Neural Network Architecture¶
The Neural Network Architecture of Entity Embed is very similar to the AutoBlock model described by Amazon.
Below is an image illustrating the steps of Entity Embed:
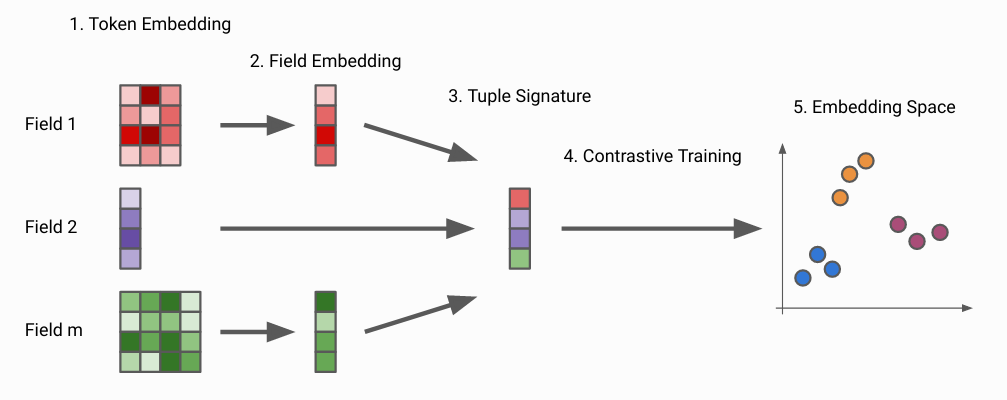
Let’s discuss each step and where Entity Embed differs from AutoBlock.
Fields¶
A single record is composed of multiple string fields. Currently only strings are supported.
Each record field can be composed of multiple tokens or a single token. The NN deals with numericalizations of the tokens of those fields.
Check entity_embed/data_utils/numericalizer.py
.
Token Embedding¶
For field types like SEMANTIC
and SEMANTIC_MULTITOKEN
, the NN receives the index of the token embedding and uses a pre-trained frozen embedding layer like fasttext (using a torchtext Vocab object ). This type of embedding approximates token strings with similar meanings.
For field types like STRING
and MULTITOKEN
, the NN receives a numericalization of the token as a 2D tensor with characters as rows and positions as columns. This is an one hot embedding that’s processed by a character-level 1D Convolutional NN as the one described on the paper Convolutional Embedding for Edit Distance. This type of CNN is useful to approximate token strings with short edit-distances.
For each field, this step outputs one or multiple token embeddings.
Check SemanticEmbedNet
and StringEmbedCNN
in entity_embed/models.py
.
Field Embedding¶
Note the previous step embedded the tokens, but a field can be composed by multiple tokens. That happens when using field types SEMANTIC_MULTITOKEN
and MULTITOKEN
.
With a sequence of token embeddings, this step uses by default a GRU with Self-Attention to summarize the multiple token embeddings into a single embedding. It’s also possible to use simple averaging of token embeddings too when using use_attention: False
in the field config.
Note that fields SEMANTIC
and STRING
don’t need this Field Embedding step, because those fields consider the whole field string as a single token.
Check MultitokenAttentionEmbed
and MultitokenAvgEmbed
in entity_embed/models.py
.
Entity Embedding¶
With the Field Embeddings from all record fields, this step takes a learned weighted average of the Field Embeddings to output a single embedding that represents the whole record.
Check EntityAvgPoolNet
in entity_embed/models.py
.
Contrastive Training¶
With the Entity Embeddings, a contrastive loss is used to approximate in the N dimensional space embeddings of records that belong to the same true cluster.
By default SupConLoss from pytorch-metric-learning is used.
Embedding Space¶
With the optimized learned embeddings, it’s possible to index them in scalable ANN indexes and perform searches. Entity Embed uses the library N2 <https://github.com/kakao/n2/> for the ANN indexes.
Check ANNEntityIndex
and ANNLinkageIndex
in entity_embed/indexes.py
.
Differences from AutoBlock¶
Here are the key differences from Entity Embed’s architecture and AutoBlock:
AutoBlock uses only fasttext to embed tokens. Entity Embed supports character-level CNNs too, enabling embeddings focused on semantic features and syntactic features.
AutoBlock uses Bi-LSTMs on field embedding, Entity Embed uses Bi-GRUs.
AutoBlock can learn multiple entity embeddings by combining different fields (multiple signatures), Entity Embed learns only a single entity embedding (learned average of all fields).
AutoBlock uses
NTXentLoss
, Entity Embed usesSupConLoss
. Both are available on pytorch-metric-learning and you can change Entity Embed to useNTXentLoss
if you wish.
As far as we know, those are the main differences. The rest of the Entity Embed’s architecture is very similar to AutoBlock.